Intelligent Control and Testing of Wheel Loaders
This project focuses on developing intelligent control strategies for wheel loaders by integrating reinforcement learning techniques with high-fidelity digital-twin simulations and scaled physical platforms.
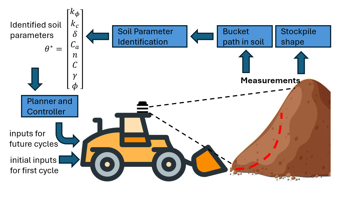
Key components include:
- Soil Parameter Identification: Estimating terrain properties to improve controller adaptability and precision in variable excavation environments.
- Reinforcement Learning Integration: Designing agents that leverage soil insights to make optimal decisions in dynamic, real-world conditions.
- Digital-Twin Simulation: A high-fidelity gravel modeling environment built using Algoryx, enabling accurate simulation of bucket–soil interactions and validation of learning-based controllers.
- Scaled Hardware Testing: A 1/14th-scale RC wheel loader is used to implement and verify control strategies, bridging the gap between theory and real-world deployment.
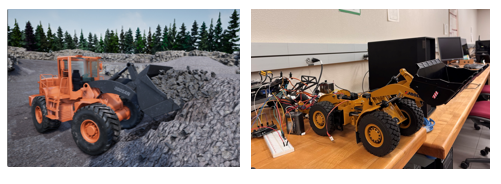
These experimental platforms ensure that control algorithms developed in simulation perform reliably when transferred to physical systems.